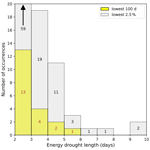
Identifying weather patterns responsible for renewable energy droughts over India
Isa Dijkstra
Hannah C. Bloomfield
Kieran M. R. Hunt
Energy systems across the globe are evolving to meet climate mitigation targets. This requires rapid reductions in fossil fuel consumption and significant uptake of renewable generation. Renewable energy sources are weather-dependent, causing production to vary at timescales from minutes to decades ahead. A consequence of this variability is that there will be periods of low renewable energy production, here termed renewable energy droughts. This energy security challenge needs to be addressed to ensure grid stability. India is chosen as a study area as it is a region that has both a large proportion of renewable generation and good subseasonal predictability.
In this study, we use synthetic wind and solar photovoltaic production timeseries, previously derived for the Indian energy grid using ERA5 reanalysis from 1979–2022, to identify historical renewable energy droughts. These are defined as periods where wind and solar potential is in the lowest 2.5 % compared to climatology. These events commonly occur from November–February, with the longest historical event being 9 d long.
We identify the weather regimes that cause the largest renewable energy droughts over India and investigate potential sources of predictability. Existing large-scale daily weather types and impact-based patterns are used to investigate the different weather patterns causing renewable energy droughts. Renewable energy droughts are caused by low seasonal wind speeds in combination with weather patterns bringing high cloud cover. These are mainly weak northeast monsoon and western disturbances.
Sources of potential subseasonal predictability are considered for the largest renewable energy droughts, including the Madden Julian Oscillation and Boreal Summer Intraseasonal Oscillation. Although both have a stronger relationship with high energy potential days, links between phases of these two oscillations and renewable energy drought days are identified. These could help to provide early warnings for challenging security of supply conditions in the future.
- Article
(5652 KB) - Full-text XML
- BibTeX
- EndNote
Global reductions in greenhouse gas emissions are required to meet national carbon targets. Decarbonisation of the electricity sector is a key mechanism to meet these targets, predominantly through the electrification of heat and transport sectors while growing the share of renewable generation (IPCC, 2021). India is the third largest primary energy consumer in the world (Jain et al., 2020), with consumption rapidly growing due to improved living conditions, urbanisation and economic development (Gulagi et al., 2017). By 2047, demand for electricity in India is expected to increase by as much as a factor of four (Lu et al., 2020). However, the country is already struggling to establish a constant and reliable power supply (Jain et al., 2020). Additionally, India is making large moves to decarbonise its energy sector. India has become one of the forerunners in the renewable energy markets around the world (Kumar and Majid, 2020). In 2022 there were 60.8 GW of installed solar power and 41.7 GW of installed wind power (Hunt and Bloomfield, 2024), However, coal still accounts for the bulk of India's primary energy supply, (Gulagi et al., 2017; Lu et al., 2020).
Beyond the current installed capacity, India has a large potential capacity for renewable energy sources, specifically wind and solar. Solar energy has immense potential in a tropical country like India, as most parts of the country receive around 300 sunny days in a year with an average of eight hours of daily sunshine (Mahtta et al., 2014). Wind power is most promising during the monsoon when there are strong westerlies along the entire length of the west coast. Recent reports found extremely large unrealised potential of both resources (Jain et al., 2020; Hunt and Bloomfield, 2024).
Increasing the amounts of renewables increases the meteorological sensitivity of the energy system, as wind and solar energy are dependent on meteorological conditions for the supply of power. An important consequence of this increased sensitivity to meteorological conditions is the possibility of renewable energy droughts occurring. Renewable energy droughts are periods when generation from renewable energy sources is unusually low. These impact grid stability and reliability of power supply (Gangopadhyay et al., 2022b) and may be the greatest risk for power systems in high renewable penetration scenarios (Matsuo et al., 2020). Understanding the frequency and severity of renewable energy droughts is important to create a secure power supply (as during times of low renewable energy production alternative – possibly carbon intensive – solutions are required), and can aid decisions regarding investment in future storage capacity (Raynaud et al., 2018). Moreover, understanding the weather patterns that are responsible for causing energy droughts is key to forecasting energy droughts in advance (Bloomfield et al., 2020). The weather patterns can also be used to plan additional capacity building, if there are regions that have the potential for high renewable energy production during energy drought days (Grams et al., 2017; Bloomfield et al., 2020).
While renewable energy droughts can have important consequences, there is no standard definition of them. Raynaud et al. (2018) characterise two types of energy droughts: Energy Production Droughts (EPDs) and Energy Supply Droughts (ESDs), where EPDs are periods with low power production and ESDs are periods with a mismatch between production and demand. Both of these definitions need a threshold under which something is considered a drought. This is generally taken as a certain percentile of wind power generation, or residual load (i.e. demand-net-renewables). This threshold is variable across studies. Ohlendorf and Schill (2020) use thresholds of 2 %, 5 % and 10 % for wind energy droughts, while Rinaldi et al. (2021) define a drought when the daily power is less than half of the 39-year daily mean for that day of the year. Beyond definitions, another consideration is choice of data type. Extreme events, by definition, occur infrequently, so long and reliable records are required. This means that metered power system data cannot be used due to the constantly evolving distribution of renewable energy sources (Cannon et al., 2017). While different studies use different definitions of energy droughts, and might use different data types, there are some common findings in studies done around the globe. The occurrence of renewable energy droughts is generally found to decrease with the severity of the drought in studies across Europe (Leahy and McKeogh, 2013; Ohlendorf and Schill, 2020; Otero et al., 2022), Africa (Seyedhashemi et al., 2021), and India (Gangopadhyay et al., 2022a). Drought frequency, duration, and intensity also tend to decrease when considering multiple energy sources or considering larger geographic areas (Handschy et al., 2017; Pryor et al., 2020; Jurasz et al., 2020; Rinaldi et al., 2021). Gangopadhyay et al. (2022a) studied renewable energy droughts in India using a stochastic weather generator to generate 5000 years of weather data to consider renewable energy droughts with large return periods. Using the stochastic weather generator, Gangopadhyay et al. (2022b) found that wind energy droughts are more intense than solar energy droughts, and they explored the role of wind-solar hybridisation in dealing with wind energy droughts. The results are regionally dependent, as they found that in South India, hybrid plants perform better than either wind or solar plants alone, while in Rajasthan, solar plants generally perform better. However, the results of Gangopadhyay et al. (2022b) are limited to only two regions of India and do not investigate the weather conditions responsible for the observed energy droughts.
Some previous work has considered the relationship between potentially stressful energy system conditions and large-scale weather patterns. Dunning et al. (2015) investigated the relationship between Indian monsoon phases, wind power and temperature-dependent demand. They found that active monsoon phases have stronger winds and therefore higher wind power generation. However, they also experience lower temperatures resulting in lower demand. The opposite is true for monsoon break phases. This opposing relationship between reduced wind power supply and increased cooling energy demand during prolonged monsoon breaks can provide a challenge to energy systems (Kulkarni et al., 2018), especially if these are combined with cloudy conditions so solar generation is low.
As well as knowing the weather conditions responsible for renewable energy droughts over India, there is a need to understand their predictability and the potential limitations of energy-based early warning systems. This paper will focus on the medium to subseasonal predictability range, as this has previously been discussed as key for energy stakeholder decision making (White et al., 2017). Das and Baidya Roy (2021) investigated the subseasonal to seasonal scale predictability of energy-relevant variables over India. They considered the variables 10 m wind speeds, incoming solar radiation, 2 m temperature and 2 m relative humidity, during April to May when energy demand is highest, and during June to September when renewable power production is higher than demand, for 1-, 2-, 3-, 4-, and 5-month lead times. Das and Baidya Roy (2021) found that overall, the skill levels were low, therefore there is great scope for using pattern-based predictability (e.g. Bloomfield et al., 2020).
Neal et al. (2020) developed a set of 30 weather patterns over India using a k-means clustering algorithm. These patterns are designed to represent country-scale precipitation variability. The weather patterns generally persist for 2–3 d, although there are occurrences of weather patterns persisting for over 20 d. As these patterns were developed for understanding precipitation variability (predominantly for landslide modelling) they have the potential to capture variations in solar radiation – and therefore solar power – well. Winds are also one of the variables used in the clustering algorithm, so there is an expectation that the patterns may also relate to wind power generation. Neal et al. (2022) reported skill in predicting these patterns from 10–15 d ahead, depending on the pattern. Patterns falling in the winter dry period regime have the highest forecast skill, and weather patterns falling into the monsoon onset and monsoon break weather regimes have the lowest forecast skill.
Beyond weather patterns, another source of predictability could come from links to modes of intraseasonal variability such as the Madden Julian Oscillation (MJO) and the Boreal Summer Intraseasonal Oscillation (BSISO). The MJO and BSISO are strongest in different seasons. As its name suggests, the BSISO dominates in boreal summer, from June to October, while the MJO dominates from December to April. November and May are transition months, and these are influenced by both the MJO and the BSISO (Kikuchi, 2021). The BSISO and MJO both have an active or convective phase which are associated with a large increase of convection and the occurrence of large and deep cloud clusters, while the opposite occurs during the inactive phase. Both the MJO and BSISO are described by eight phases based on their propagation along the Equator. Each of these phases has a different signature over India with potential impacts on the energy system through changes in solar power generation (associated with convective anomalies) and changes in wind power generation (through changes in the near-surface wind speeds). Subseasonal to seasonal models can make skilful forecasts of the MJO at lead times of two to four weeks ahead; with the BSISO having similar predictability (Vitart, 2017). Thus, if the relationships between these intraseasonal models and potential low energy production days are shown to be consistent, this could give an indication of the probability of these events occurring up to a month ahead with higher confidence than using forecasts of gridded meteorological variables.
Another approach to considering the subseasonal predictability of energy-relevant variables was used over Europe by (Bloomfield et al., 2020), who developed Targeted Circulation Types (TCTs). Rather than using already established weather patterns, TCTs characterise the large-scale circulation patterns that are of most interest to the electricity grid by clustering energy-relevant variables. While TCTs are better at characterising variability of extremes (e.g., the probability of demand-net-renewables being in the upper 10 % of the climatological distribution), they did not lead to significantly better subseasonal forecasts than traditional patterns (Bloomfield et al., 2021).
Despite their rapidly increasing investment in renewables, there has been little work exploring the likelihood of renewable energy droughts in India, the weather patterns responsible, and how predictable they are. Therefore, this study has the following aims:
-
To identify weather patterns that are associated with Indian combined wind and solar energy droughts.
-
To define a set of impact-based patterns for forecasting Indian renewable energy.
-
To explore the potential predictability at subseasonal timescales of Indian combined wind and solar energy droughts.
This study looks into the different weather patterns associated with renewable energy droughts in India, and considers potential sources of predictability. Section 2 describes the data and methods used to analyse the energy droughts in this study and relevant meteorological patterns used to asses the sources of potential predictability. This study is laid out as follows. We characterise renewable energy droughts from wind and solar power generation (Sect. 3.1), their relationship to traditional weather patterns (Sect. 3.2), and the potential for impact-based patterns to characterise renewable energy droughts (Sect. 3.3). We then discuss the implications of this work (Sect. 4), and conclude with the key findings and possibilities for future work (Sect. 5).
2.1 Energy drought definitions
Energy droughts are defined here following the energy production droughts described in Raynaud et al. (2018), where a low production period is a contiguous sequence of days during which the combined wind and solar power generation is below a given low-production threshold (in our case the lowest 2.5 % of days). A key difference from Raynaud et al. (2018) is we maintain the single-day events as these are useful for the daily weather-pattern analysis conducted in Sect. 3.2. We consider only combined wind and solar droughts in this study, but the individual cases (i.e. wind power only or solar power only) are sometimes commented on to add context to the discussion. We note hydropower is not included in the renewable energy generation totals in this study.
Initially the 100 d with the lowest synthetic energy production are taken as the renewable energy drought days. This value is chosen because it represents extremely low energy production (lower than the 1st percentile), while still having enough data to conduct analysis. When considering the timing of renewable energy droughts associated with different teleconnections the lowest 2.5 % of synthetic energy production data is used to provide enough data when the drought days are split over the eight MJO or BSISO phases.
2.2 Meteorological datasets
The ERA5 reanalysis (Hersbach et al., 2020) is used for all meteorological variables in this study. ERA5 is produced by the European Centre for Medium-Range Weather Forecasts (ECMWF) and is their fifth generation of atmospheric reanalyses. The period covered by ERA5 is 1940 to the present day, and within this study the period of 1979–2022 has been used. ERA5 data is produced and archived as spectral coefficients or on a reduced Gaussian grid, which has quasi-uniform spacing over the globe. It therefore has a horizontal resolution of approximately 31 km over India, with 137 vertical levels (Hersbach et al., 2020). The following surface-level variables are used at hourly timescales: mean downward shortwave radiation flux, zonal and meridional wind speeds at 10 and 100 m and temperatures at 2 m. These are used to drive the wind power and solar power models described in Sect. 2.3.
Only data from one daily time step are used for the surface variables composites in Sect. 3.2. The times used are 09:00 UTC for mean downward shortwave radiation (as this is the middle of the day in India and thus has maximum incoming solar radiation), and 12UTC for the wind variables (as India experiences a strong diurnal cycle in near-surface wind speeds and this is a time of day that is representative of the median wind speeds). The anomaly fields (e.g. x′) for the low energy potential days are calculated by subtracting a weighted monthly mean field – e.g., for 7 June, we would subtract the mean value for all Junes from 1979–2022 – from the composite mean of the low energy potential days (e.g. ) as shown in the following equation:
2.3 Wind and solar power data
A timeseries of hourly wind and solar power capacity factors are used from Hunt and Bloomfield (2024), spanning 1979 to 2022. The wind power capacity factors are calculated with a physical model, where hourly gridded 100 m wind speeds over India are passed through a representative power curve to convert from wind speed to capacity factor. It is assumed that in each grid box the most suitable turbines are installed, which are those that maximise synthetic power production (see Sect. 2.5 of Hunt and Bloomfield, 2024 for details). These capacity factors are then converted into national generation totals in GW by weighting by the locations of installed wind generation in 2022. Installed wind power generation in 2022 is largely concentrated in the western edge of the Indian peninsula, with highest densities along the Western Ghats and in the Rann of Kutch (Gujarat).
Timeseries of India-averaged solar power capacity factors (SP_CF) are created as in Bloomfield et al. (2020). Hourly gridded 2 m temperatures and incoming shortwave irradiance are used as the meteorological input to the following equation:
where G(t) is the incoming (i.e. downward) shortwave radiation at the surface, Gref=1000 W m−2, T(t) is the environmental 2 m temperature (with which the photovoltaic cell is assumed to be in equilibrium), and η is the relative efficiency, given by:
where ηref=0.9, βref=0.042, and Tref=25 °C.
These gridded capacity factors are then aggregated using the weighted mean, where the weights are the known locations of solar power generation. From this we get a timeseries of synthetic solar production over the whole of India in GW. Solar power installations are relatively evenly spread across India with much of the south having some coverage. The two models verify well against measured data with an R-statistic greater than 0.9 (see Sect. 2.5 of Hunt and Bloomfield, 2024 for full verification). We note that when energy production is referred to in the remained of the study, this is referring to the reanalysis-driven wind and solar power data described in this section.
2.4 Indian weather patterns
To consider the types of weather patterns responsible for renewable energy droughts, the 30 weather patterns derived by Neal et al. (2022) are used. These patterns are designed to represent precipitation variability and include all the main monsoonal and non-monsoonal circulation types that occur throughout the year. They are created using a non-hierarchical k-means clustering algorithm applied to ERA-Interim reanalysis data from 1979 to 2016 (Dee et al., 2011). The variables used in the clustering were pressure at mean sea-level, and zonal and meridional winds at 10 m, 925 hPa, and 850 hPa wind. The moisture information is implicitly included in these weather regimes through the wind vectors because moisture is directly related to wind direction over India. 192 sets of weather patterns were created using a hierarchical clustering methodology for various geographical areas and combinations of meteorological variables. The set of weather pattern that were retained were those that best represented precipitation variability using an explained variation score. This score measures how well a daily precipitation series can be reconstructed based on knowledge only of the weather pattern classification (Beck et al., 2013). Pattern numbers are freely available for each day from 1979–2016 and can be downloaded from https://doi.org/10.1594/PANGAEA.902030 (Neal et al., 2019). This timeseries was used to determine the relationship between weather patterns and energy drought days in Sect. 3.2.1.
2.5 Teleconnection indices
Daily data on MJO and BSISO phase and amplitude are used to understand their behaviour during renewable energy droughts. MJO phase and amplitude are based empirical orthogonal functions of zonal wind at 850 and 200 hPa, and outgoing longwave radiation in the near-equatorial region (Wheeler and Hendon, 2004). The MJO dataset is freely available from the Australian Government Bureau of Meteorology at http://www.bom.gov.au/climate/mjo/ (last access: 3 May 2023).
BSISO data is also based on a pair of empirical orthogonal functions, but these are created using only outgoing longwave radiation and zonal wind at 850 hPa, and are taken over the region 10° S–40° N, 40–160° E (Lee et al., 2013). BSISO data is obtained from the International Pacific Research Centre at the University of Hawaii at https://iprc.soest.hawaii.edu/users/kazuyosh/ISO_index/data/BSISO_25-90bpfil_pc.extension.txt (last access: 3 May 2023). Following convention, only days with an MJO or BSISO amplitude of higher than 1 are considered, as amplitudes below 1 indicate an insignificant MJO or BSISO event (e.g. Kikuchi, 2021).
2.6 Impact-based patterns
Impact-based patterns are created using the large-scale meteorological variables present during low energy production days over the region [5–40° N, 60–100° E]. Clusters are computed by applying k-means to 850 hPa u, 850 hPa v, and surface shortwave radiation flux on days in which total modelled energy production is in the bottom 2.5 %. Data are taken from ERA5, with winds masked where the orography is higher than 1500 m (i.e. approximately 850 hPa). An elbow method was used to determine the optimal cluster number of three. Sensitivity analysis was conducted on the most appropriate variables to use to capture the behaviour of renewable energy droughts (e.g., using u and v component winds from 500, 250 hPa and using relative humidity or cloud cover data to represent potential convection). However, all cluster sets look qualitatively similar, and lead to 3 as an optimal number.
3.1 Renewable energy droughts
Over India, renewable energy droughts are most common from November–February, with some events happening as early as October (Fig. 1). The lowest 100 d of combined wind and solar generation predominantly occur between December and February. However, when relaxing this definition to consider the lowest 2.5 % of renewable energy generation the period of interest extends from late August to early March (Fig. 1). During the post-monsoon period (October–November) the average renewable generation is 14.3 GW. However, of this an average of 10 GW is solar generation, with wind energy production often close to zero after the withdrawal of the summer monsoon but before the onset of the northeast winter monsoon. This means that the renewable energy drought days are commonly driven by variability in wind energy production. 73 % of the renewable energy drought days occur on days on which there is no separate wind or solar energy drought if the two renewable sources are considered in isolation. This indicates that the majority of renewable energy drought days occur due to a combination of relatively low wind and solar, while the wind or solar power production is not extremely low by itself. A similar result is found considering the lowest 2.5 % of combined energy production days, and for these days an even larger proportion (84.1 %) are found to not overlap with an individual wind or solar energy drought days.
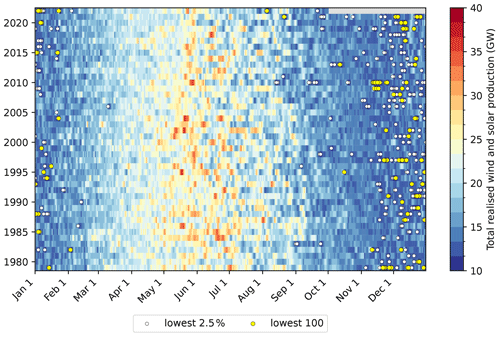
Figure 1Total realised wind and solar energy production across India from 1979 to 2022 assuming the 2022 renewable energy capacity had been installed for the whole time period. Small white dots mark days with energy production in the lowest 2.5 %. Large yellow dots mark the 100 d with lowest energy production.
When considering the lowest 100 d of renewable energy droughts, 20 of these days are multi-day events, i.e. occur in consecutive periods of at least 2 d. The longest period in this extreme energy drought definition lasts 5 d (see Fig. 2).
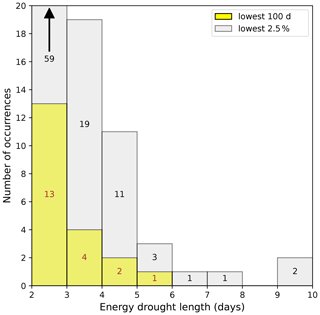
Figure 2Distribution of length of energy droughts in days for the 100 lowest energy production days (yellow) and the lowest 2.5 % energy production days (grey).
Extending this to include days in the bottom 2.5 %, the longest renewable energy droughts last 9 d. This occurred twice: once in December 1991 and once in November 2010. Occurring twice within the 43-year period studied here gives such events an approximate return period of twenty years. These prolonged periods of critically low generation would put a large strain on the power system from both a energy system security (i.e., keeping the lights on) and carbon intensity (i.e., meeting net-zero targets) perspective. Within this definition there are 96 events which are multi-day events, showing this is a relatively frequent problem (of the order of two each year) multi-day events. The potential for a week-long energy drought is particularly important for future power systems with even higher proportions of renewables, and for such systems it will be vital to mitigate this using wind and solar power complementarity (Jurasz et al., 2020; Hunt and Bloomfield, 2024).
3.2 Relationship between energy droughts and traditional weather patterns
3.2.1 The 30 Indian weather patterns
Figure 3 shows the occurrence frequency of each of the 30 weather patterns from Neal et al. (2020) during renewable energy droughts when compared to their climatological frequency. This climatological comparison is important as the patterns vary considerably in seasonal and total frequency (Neal et al., 2020). The weather patterns which are most associated with energy droughts are the winter dry period (patterns 7 and 9, which both have very low winds over the West coast of India), the retreating monsoon (pattern 1, low wind speeds over the North-West of India), and western disturbances (pattern 27, low winds over the South of India and high precipitation in the North). Pattern 1 mainly occurs from October to December, with patterns 7, 9 and 27 being more common from December to April (Neal et al., 2020), Thus, all these patterns occur mainly in the seasons that the energy drought days occur (see Fig. 1). Out of these four weather patterns, pattern 1 has the highest average persistence of 3.5 d, while patterns 7 and 9 have an average persistence of 2 d, and pattern 27 of 1.5 d (Neal et al., 2020). Another factor to note is that when a day is classified as pattern 27, there is a larger than 30 % probability that the next day will be pattern 7 (Neal et al., 2022). This means that energy droughts that start with pattern 1 or pattern 27 are likely to persist for longer. When analysing the renewable energy production droughts of 3 d in length the weather patterns associated with the winter dry period (patterns 7, 9 and 20, not shown) are three which occur most commonly compared to climatology. This suggests that moderately different weather conditions are responsible for the extended duration events compared to the single day events. The data volumes are however very small for this analysis (approximately 100 d divided between 30 weather patterns) so we would suggest the use of longer climate simulations for robust confirmation of these results.
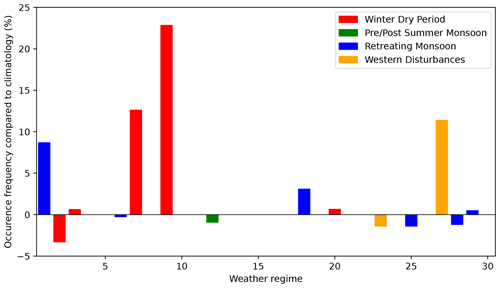
Figure 3Occurrence frequency of weather patterns defined by Neal et al. (2020) on the 100 lowest energy production days minus the climatological occurrence frequency. Weather patterns that did not have any low energy days have been set to zero.
While these patterns do not guarantee the presence of energy droughts, they may still serve as useful indicators in longer-range forecasts, particularly if the weather pattern forecasts (which have skill out to 10–15 d depending on the pattern; Neal et al., 2022) are more skillful than forecasts of gridded surface variables (Das and Baidya Roy, 2021).
3.2.2 The MJO and BSISO
The MJO and BSISO have strong seasonality, with the MJO predominantly being active between December and April, and the BSISO between June and September (Kikuchi, 2021). Given the tendency for renewable energy drought days to occur between November and February (Fig. 1), we would therefore expect a stronger relationship with the MJO and energy drought days than with the BSISO. Figure 4 shows composites of anomalous 100 m wind vectors and downwelling solar radiation averaged over each MJO phase. We note here a phase is a distinct stage within the process of the movement of a region of tropical convection from the Indian ocean to the West Pacific. During phases 1 and 8, negative radiation anomalies (and therefore high cloud cover) are seen over central and northern India. However, these are generally accompanied by relatively windy conditions over south India. Phases 2 and 3 show, on average, relatively windy and sunny conditions except for in the far south of India. In phases 4 to 6 there are positive shortwave radiation anomalies over northwest India (where many solar panels are located) with positive anomalies seen in south India for phase 7.
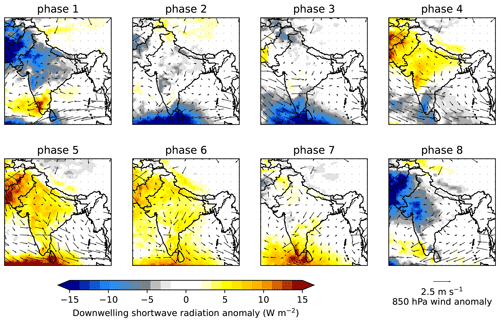
Figure 4Downwelling shortwave radiation anomalies and 850 hPa wind anomalies over India for each phase of the Madden Julian Oscillation (MJO). Averaged over December–April 1979–2022, showing the movement of convective anomalies. Positive cloud cover anomalies can be seen through negative radiation anomalies and vice versa. Anomalies are computed relative to the respective climatological monthly means. Data are from ERA5, with winds masked where the orography is higher than 1500 m.
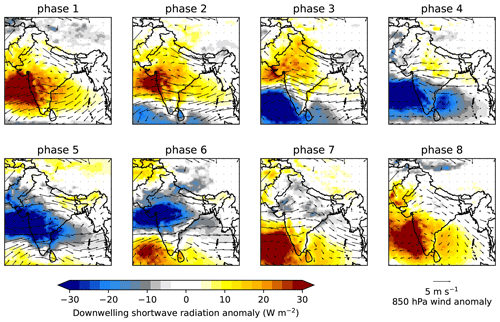
Figure 5Downwelling shortwave radiation anomalies and 850 hPa wind anomalies over India for each phase of the BSISO. Averaged over June–September 1979–2022, showing the movement of convective anomalies. Positive cloud cover anomalies can be seen through negative radiation anomalies and vice versa. Anomalies are computed relative to the respective climatological monthly means. Data are from ERA5, with winds masked where the orography is higher than 1500 m. Note the colour and quiver scales differ from Fig. 5.
Figure 5 shows composites of anomalous 100 m wind vectors and downwelling solar radiation during each BSISO phase. The lowest solar radiation anomalies are seen in phases 3–6 where convection is enhanced over much of India, firstly impacting the south of India (phases 3–4) and then moving to central India (phases 5–6). However, this convection itself leads to increased wind power generation along the western coast of the peninsula, which is the region with the highest installed wind capacity. Positive solar radiation anomalies are seen in phases 7 and 8 over southwest India, which then propagate into central India in phases 1 and 2. Of these four phases the lowest wind speeds are seen in Phase 8 (Fig. 5).
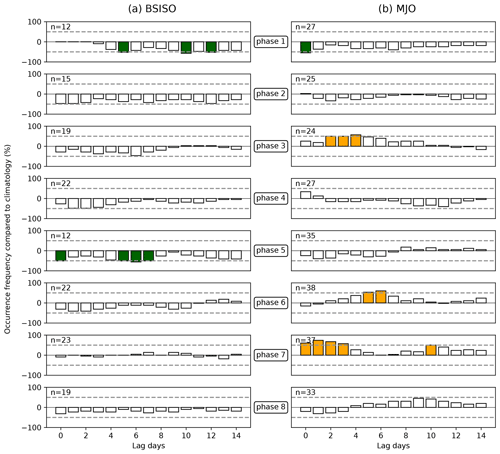
Figure 6Occurrence frequency of each BSISO phase (a) and MJO phase (b) on low energy production days and the 14 d beforehand compared to climatology. The numbers indicate percentage difference from climatology, so −100 means no occurrence of this phase, and 100 means that this phase occurs twice as frequent than its climatological mean. Bars are colored in if they are higher than 50 % or lower than −50 % as in Cassou (2008).
Due to the complementarity between wind and solar power during periods of strong convection, especially during the summer monsoon, there are not particularly strong links between MJO or BSISO phase and the occurrence of renewable energy droughts. Figures 4 and 5 suggest that the renewable energy drought days are more likely to be associated with phases 1 and 8 of the MJO and phases 4–6 of the BSISO. However, previous work has shown that the weather conditions during renewable energy drought days do not necessarily resemble the features from composites over the weather patterns (Wiel et al., 2019). In Fig. 6, we therefore show the occurrence frequency of renewable energy drought during each phase of the BSISO and MJO. Given the predictability of the MJO out to two weeks ahead and beyond (Vitart, 2017), a lag is also included to see if this relationship could provide information on the potential predictability of the patterns. Figure 6 shows that a presence of a strong BSISO of any phase is unlikely to lead to an energy drought. This is logical as generally the BSISO is strongest during the summer monsoon, a period of typically high renewable energy production, and energy droughts tend to occur in winter. The BSISO does, however, have a significant impact on the occurrence of energy surplus events (Fig. A1).
Despite the negative solar radiation anomalies in north India in phase 1 of the MJO, the relatively strong winds and positive anomalies in the south mean that India is up to 50 % less likely than average to experience a renewable energy drought. Phase 3 however, shows increased probabilities of an energy drought occurring, particularly at a lead time of 3–5 d, as the anomalous westerlies along the west coast act to weaken the climatological easterlies. Interestingly, phases 6 and 7 – which on average show positive radiation anomalies, and weak wind anomalies along the west coast – are associated with an increased risk of energy drought. This emphasises why the mean composite behavior from Fig. 4 does not necessarily give a measure of renewable energy drought day behaviour. Comparing Figs. 4 and 6, we see that low solar radiation anomalies in the south of India are particularly important for the occurrence of renewable energy droughts. However, more bespoke classifications are needed to confirm this.
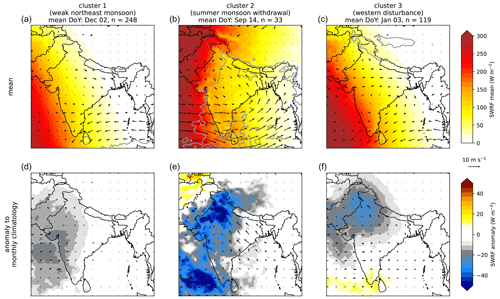
Figure 7Composites of 850 hPa winds and surface shortwave radiation (SWRF) for three impact-based clusters. (a–c) Cluster mean 850 hPa winds and SWRF. (d–f) The same fields are shown as anomalies to their respective climatological monthly means. For each cluster, the title shows a mean day-of-year, determined through circular statistics; the cluster population; and, in parentheses, the circulation type most associated with the cluster.
Although the results from Fig. 7 do not suggest a particularly robust link between the MJO, BSISO and renewable energy droughts, Fig. A1 shows that these patterns are potentially useful to users interested in excess renewable generation (here considering the top 2.5 % of renewable energy generation) and therefore possible grid-induced curtailment. This is perhaps surprising for the MJO, given the seasonality of the pattern making it most common in winter. However while mean production is low in winter, particularly active MJO events can drive strong westerly wind bursts over the south of the peninsula (Joseph et al., 2009; Liang et al., 2021), briefly causing a surge in wind production.
3.3 Impact-based patterns
As we have shown, many traditional weather patterns are not strongly associated with renewable energy droughts (Figs. 3 to 6). This suggests that if renewable energy droughts are to be accurately predicted at sub-seasonal lead times, a more bespoke solution is needed.
We now perform impact-based clustering to isolate groups of weather conditions present during energy drought days. Figure 7 shows the renewable energy drought days are caused by three distinct weather patterns, which tend to happen at a set time of year. These weather patterns can be interpreted, from left to right, as a northeast monsoon (centred in early December, comprising 62 % of the total), a summer monsoon withdrawal (a small group, centered in September, comprising 8 % of the total), and a western disturbance (most common in early January, comprising 30 % of the total).
However, we can advance on our earlier analysis by identifying how these particular patterns, leading to energy droughts, differ from the generic patterns of Neal et al. (2020). Firstly, we note that the northeast monsoon is unusually weak, evidenced by anomalous westerlies along the west coast. This results in very low wind production, but also allows convection to build up along the coast, where it would usually be suppressed by dry air blown off the peninsula by prevailing easterlies, and thus solar production is also lower than the (already low) winter average. Secondly, the summer monsoon withdrawal usually represents a transition from strong westerlies along the west coast to very weak winds, and we see that in this composite, as the westerlies are confined to the southern part of the peninsula. However, a late burst of southeasterlies, perhaps triggered by a low-pressure system or active BSISO, pushes moist air over northwest India (a region of high installed solar capacity), where deep convection significantly reduces solar production. This pattern, though comparatively rare, is potentially very important, as energy demand is much higher in September, when the conditions are hot and humid, than in the winter months. Thirdly, while we would expect increased cloud cover associated with the passage of a winter western disturbance, this composite suggests more widespread cloud cover – especially to the south, where it reaches into central India and the Gujarat coast – significantly reducing insolation over a region of large installed solar capacity. Of additional note, the surface winds associated with this composite western disturbance are quite weak. Combined, these characteristics suggest a weak western disturbance passing unusually far south, and thus close to the Arabian Sea moisture source.
These patterns are thus consistent with our earlier analysis based on the thirty weather patterns (Fig. 3), but offer much finer detail on what variations within those patterns lead to energy droughts. Renewable energy droughts occurring when the northeast monsoon is weak are driven by very low wind energy production and moderately low solar energy production. Conversely those associated with western disturbances and summer monsoon withdrawals are related to very low solar energy production. These contrasting conditions depending on the time of year are interesting, as they show a potential seasonal cycle in the key drivers of renewable energy droughts. This is quite different to the weather conditions previously discussed for Europe, which tend to be predominantly driven by winter anticyclones (Bloomfield et al., 2020).
Both wind and solar energy production in India have strong seasonal cycles, which means that almost all renewable energy droughts occur during the post-monsoon and winter season. As the weather patterns experienced over India are strongly determined by the season, this limits the range of possible weather patterns that could be associated energy drought days. The analysis of combined wind and solar drought days is particularly interesting, due to the generally good complementarity between the resources at national scale (see Hunt and Bloomfield, 2024. Although it has previously been noted that the complementarity is better from May–October than during the winter, when the largest number of renewable energy droughts are found (Gangopadhyay et al., 2022a).
The frequency of energy droughts decreases with the length of the energy drought, which is consistent with previous studies done over other regions (Otero et al., 2022; Ohlendorf and Schill, 2020). The longest events seen are 9 d in the reanalysis period. These events have a return period of approximately 20 years (as we see two of them in the 43 year period, although we note the uncertainty on this return period is very high with this many years of data). This is frequent enough that it would be important to consider for energy providers in India.
Combined wind and solar energy drought days were found to mostly be independent of individual solar energy or wind energy drought days, indicating that they are caused by different weather patterns. This could be low solar generation on a moderate wind day (e.g. the western disturbance or summer monsoon withdrawal patterns) or a low wind energy day with moderate solar power generation (e.g., the weak northeastern monsoon pattern). While these patterns are the patterns that would cause energy drought days with the current renewable energy capacity, we note that India is strongly investing in solar energy. This will change the mix of solar and wind energy, and might result in different weather patterns causing energy droughts.
Although the MJO and BSISO are predictable up to two to four weeks out (Lee et al., 2015; Vitart, 2017), it is unlikely they could give an indication of the probability of an energy drought occurring at the seasonal to subseasonal timescale due to their generally weak relationship with renewable energy drought events. They may be useful, however, in predicting energy surplus events.
This study focused only on energy production drought, but analysis into weather patterns causing energy supply droughts (i.e., also including electricity demand) would be a useful extension of the analysis.
Recently, Das and Roy (2024) showed that the JRA55 reanalysis (Kobayashi et al., 2015) provides the best representation of near-surface wind speeds over India compared to gridded observations. In the key regions of interest for wind power production (the West coast, East coast and Interior peninsula) the wind speed distributions in JRA55 are closest to the observations with ERA5 performing second best out of the six reanalysis products that were compared (including the Indian Monsoon Data Assimilation and Analysis (IMDAA) regional reanalysis; Rani et al., 2021). ERA5 has been used in this study as a demonstration of the relationships between various large-scale classifications and wind and solar power generation, and due to it's continual updates. However, future work could consider repeating this work with JRA55 to confirm the accuracy of the findings.
Investment in renewable energy in India is currently largely skewed towards solar energy. As this would change the ratio between solar and wind energy capacity, the weather patterns that have the potential to cause energy droughts now might not do so in the future. Therefore, analysis into which weather patterns would cause energy droughts with increased solar capacity is necessary, as this will give insight into the weather patterns that will cause energy droughts in the future.
This study has investigated the meteorological drivers of renewable energy droughts over India under and extreme definition (lowest 100 d of combined wind and solar power generation) and the lowest 2.5 % of solar generation, similar to Raynaud et al. (2018). The key results are given below:
-
Renewable energy droughts are most common from November to February (Fig. 1) and are often multi-day events. The longest event seen is 9 d (Fig. 2) which happens twice during the 42 year period.
-
There is very little overlap between the days which would classify as a wind or solar energy drought and a renewable energy drought. This highlights the complementarity between the two renewables, and that the renewable energy drought days are driven by moderate generation by one of the two renewables, and low generation by the other.
-
Renewable energy droughts happen during particular weather patterns, such as a winter dry period, or a western disturbance, which have significantly increased occurrence frequency compared to climatology (see Fig. 3). These patterns are predictable 10–15 d ahead (Neal et al., 2020) suggesting they could support sub-seasonal predictability of renewable energy droughts.
-
There are no strong relationships between the MJO or BSISO and renewable energy droughts (Figs. 4 to 6) although these patterns may be useful if interested in renewable energy surpluses (Fig. A1) or average renewable conditions (Figs. 4 and 5) given their good subseasonal predictability (Lee et al., 2015; Vitart, 2017).
-
Impact-based forecasting patterns highlight the different conditions that may cause a renewable energy drought including a weak northeastern monsoon pattern, summer monsoon withdrawal and western disturbance (Fig. 7).
Given the high explanatory power of these impact-based patterns, future work will investigate their medium-range to subseasonal predictability to compare directly to the MJO and BSISO. It is interesting to understand if there is a trade off between predictability and links to useful impacts (as discussed in Bloomfield et al., 2020).
This work is highly relevant for forecasters in India (or similar regions) looking to understand the critical weather conditions for energy system security. We note that due to rapid decarbonisation these patterns need to be revisited every ∼ 5 years to make sure that they are still relevant to the energy system they are describing.
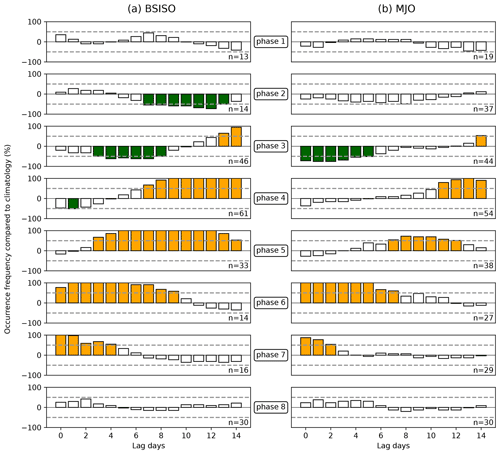
Figure A1Occurrence frequency of each BSISO phase (a) and MJO phase (b) on high energy production days and the 14 d beforehand compared to climatology. The numbers indicate percentage difference from climatology, so −100 means no occurrence of this phase, and 100 means that this phase occurs twice as frequent than its climatological mean. Bars are colored in if they are higher than 50 % or lower than −50 % as in Cassou (2008).
Analysis code from this project is stored in the following GitHub respository and at Zenodo: https://github.com/kieranmrhunt/india-renewable (last access: 3 May 2023) and https://doi.org/10.5281/zenodo.14265562 (Hunt, 2024).
The wind and solar power timeseries used in this project are taken from https://doi.org/10.5281/zenodo.7824872 (Hunt and Bloomfield, 2023). The ERA5 reanalysis data was downloaded from the Coppernicus Climate Data Store (https://doi.org/10.24381/cds.adbb2d47, Hersbach et al., 2023).
Project conceptualisation was performed by HCB and KMRH. ID was a masters student at University of Reading who performed the bulk of the analysis during this project. This was then finished off by HCB and KMRH on completion of the project and they jointly wrote the paper.
The contact author has declared that none of the authors has any competing interests.
Publisher's note: Copernicus Publications remains neutral with regard to jurisdictional claims made in the text, published maps, institutional affiliations, or any other geographical representation in this paper. While Copernicus Publications makes every effort to include appropriate place names, the final responsibility lies with the authors.
This article is part of the special issue “European Geosciences Union General Assembly 2024, EGU Division Energy, Resources & Environment (ERE)”. It is a result of the EGU General Assembly 2024, Vienna, Austria, 14-19 April 2024.
Kieran M. R. Hunt is supported by a NERC Independent Research Fellowship (MITRE; grant no. NE/W007924/1). Hannah C. Bloomfield is funded by a Newcastle University Academic Track Fellowship.
This paper was edited by Sonja Martens and reviewed by Somnath Baidya Roy and one anonymous referee.
Beck, C., Philipp, A., and Streicher, F.: The effect of domain size on the relationship between circulation type classifications and surface climate, Int. J. Climatol., 36, 2692–2709, https://doi.org/10.1002/joc.3688, 2013. a
Bloomfield, H. C., Brayshaw, D. J., and Charlton-Perez, A. J.: Characterizing the winter meteorological drivers of the European electricity system using targeted circulation types, Meteorol. Appl., 27, e1858, https://doi.org/10.1002/met.1858, 2020. a, b, c, d, e, f, g
Bloomfield, H. C., Brayshaw, D. J., Gonzalez, P. L., and Charlton-Perez, A.: Pattern-based conditioning enhances sub-seasonal prediction skill of European national energy variables, Meteorol. Appl., 28, e2018, https://doi.org/10.1002/met.2018, 2021. a
Cannon, D., Methven, J., Brayshaw, D., and Drew, D.: Determining the bounds of skilful forecast range for probabilistic prediction of system-wide wind power generation, Meteorol. Z., 26, 239–252, https://doi.org/10.1127/metz/2016/0751, 2017. a
Cassou, C.: Intraseasonal interaction between the Madden–Julian Oscillation and the North Atlantic Oscillation, Nature, 455, 523–527, https://doi.org/10.1038/nature07286, 2008. a, b
Das, A. and Baidya Roy, S.: Evaluation of subseasonal to seasonal forecasts over India for renewable energy applications, Adv. Geosci., 56, 89–96, https://doi.org/10.5194/adgeo-56-89-2021, 2021. a, b, c
Das, A. and Roy, S. B.: JRA55 is the best reanalysis representing observed near-surface wind speeds over India, Atmos. Res., 297, 107111, https://doi.org/10.1016/j.atmosres.2023.107111, 2024. a
Dee, D. P., Uppala, S. M., Simmons, A. J., Berrisford, P., Poli, P., Kobayashi, S., Andrae, U., Balmaseda, M. A., Balsamo, G., Bauer, P., Bechtold, P., Beljaars, A. C. M., van de Berg, L., Bidlot, J., Bormann, N., Delsol, C., Dragani, R., Fuentes, M., Geer, A. J., Haimberger, L., Healy, S. B., Hersbach, H., Hólm, E. V., Isaksen, L., Kållberg, P., Köhler, M., Matricardi, M., McNally, A. P., Monge-Sanz, B. M., Morcrette, J.-J., Park, B.-K., Peubey, C., de Rosnay, P., Tavolato, C., Thépaut, J.-N., and Vitart, F.: The ERA-Interim reanalysis: configuration and performance of the data assimilation system, Q. J. Roy. Meteor. Soc., 137, 553–597, https://doi.org/10.1002/qj.828, 2011. a
Dunning, C. M., Turner, A. G., and Brayshaw, D. J.: The impact of monsoon intraseasonal variability on renewable power generation in India, Environ. Res. Lett., 10, 064002, https://doi.org/10.1088/1748-9326/10/6/064002, 2015. a
Gangopadhyay, A., Seshadri, A. K., Sparks, N. J., and Toumi, R.: The role of wind-solar hybrid plants in mitigating renewable energy-droughts, Renew. Energ., 194, 926–937, https://doi.org/10.1016/j.renene.2022.05.122, 2022a. a, b, c
Gangopadhyay, A., Sparks, N., Toumi, R., and Seshadri, A.: Risk assessment of wind droughts over India, Curr. Sci., 122, 1145–1153, https://doi.org/10.18520/cs/v122/i10/1145-1153, 2022b. a, b, c
Grams, C. M., Beerli, R., Pfenninger, S., Staffell, I., and Wernli, H.: Balancing Europe's wind-power output through spatial deployment informed by weather regimes, Nat. Clim. Change, 7, 557–562, https://doi.org/10.1038/nclimate3338, 2017. a
Gulagi, A., Bogdanov, D., and Breyer, C.: The Demand For Storage Technologies In Energy Transition Pathways Towards 100 % Renewable Energy For India, Enrgy. Proced., 135, 37–50, https://doi.org/10.1016/j.egypro.2017.09.485, 2017. a, b
Handschy, M. A., Rose, S., and Apt, J.: Is it always windy somewhere? Occurrence of low-wind-power events over large areas, Renew. Energ., 101, 1124–1130, https://doi.org/10.1016/j.renene.2016.10.004, 2017. a
Hersbach, H., Bell, B., Berrisford, P., Hirahara, S., Horányi, A., Muñoz Sabater, J., Nicolas, J., Peubey, C., Radu, R., Schepers, D., Simmons, A., Soci, C., Abdalla, S., Abellan, X., Balsamo, G., Bechtold, P., Biavati, G., Bidlot, J., Bonavita, M., and Thépaut, J.-N.: The ERA5 global reanalysis, Q. J. Roy. Meteor. Soc., 146, 1999–2049, https://doi.org/10.1002/qj.3803, 2020. a, b
Hersbach, H., Bell, B., Berrisford, P., Biavati, G., Horányi, A., Muñoz Sabater, J., Nicolas, J., Peubey, C., Radu, R., Rozum, I., Schepers, D., Simmons, A., Soci, C., Dee, D., and Thépaut, J.-N.: ERA5 hourly data on single levels from 1940 to present, Copernicus Climate Change Service (C3S) Climate Data Store (CDS) [data set], https://doi.org/10.24381/cds.adbb2d47, 2023. a
Hunt, K.: kieranmrhunt/india-renewable: Renewable Energy for India (v1.0), Zenodo [code], https://doi.org/10.5281/zenodo.14265562, 2024. a
Hunt, K. and Bloomfield, H.: Historical and modelled renewable energy production for India (v0.1), Zenodo [data set], https://doi.org/10.5281/zenodo.7824872, 2023. a
Hunt, K. M. R. and Bloomfield, H.: Quantifying renewable energy potential and realised capacity in India: opportunities and challenges, Meteorol. Appl., 31, e2196, https://doi.org/10.1002/met.2196, 2024. a, b, c, d, e, f, g
IPCC: Summary for Policymakers, in: Climate Change 2021: The Physical Science Basis. Contribution of Working Group I to the Sixth Assessment Report of the Intergovernmental Panel on Climate Change, edited by: Masson-Delmotte, V., Zhai, P., Pirani, A., Connors, S. L., Péan, C., Berger, S., Caud, N., Chen, Y., Goldfarb, L., Gomis, M. I., Huang, M., Leitzell, K., Lonnoy, E., Matthews, J. B. R., Maycock, T. K., Waterfield, T., Yelekçi, O., Yu, R., and Zhou, B., Cambridge University Press, Cambridge, United Kingdom and New York, NY, USA, 3−-32, https://doi.org/10.1017/9781009157896.001, 2021. a
Jain, A., Das, P., Yamujala, S., Bhakar, R., and Mathur, J.: Resource potential and variability assessment of solar and wind energy in India, Energy, 211, 118993, https://doi.org/10.1016/j.energy.2020.118993, 2020. a, b, c
Joseph, S., Sahai, A., and Goswami, B. N.: Eastward propagating MJO during boreal summer and Indian monsoon droughts, Clim. Dynam., 32, 1139–1153, 2009. a
Jurasz, J., Canales, F. A., Kies, A., Guezgouz, M., and Beluco, A.: A review on the complementarity of renewable energy sources: Concept, metrics, application and future research directions, Sol. Energy, 195, 703–724, https://doi.org/10.1016/j.solener.2019.11.087, 2020. a, b
Kikuchi, K.: The Boreal Summer Intraseasonal Oscillation (BSISO): A Review, J. Meteorol. Soc. Jpn. Ser. II, 99, 933–972, https://doi.org/10.2151/jmsj.2021-045, 2021. a, b, c
Kobayashi, S., Ota, Y., Harada, Y., Ebita, A., Moriya, M., Onoda, H., Onogi, K., Kamahori, H., Kobayashi, C., Endo, H., Miyaka, K., and Takahashi, K.: The JRA-55 reanalysis: General specifications and basic characteristics, J. Meteorol. Soc. Jpn. Ser. II, 93, 5–48, https://doi.org/10.1186/s13705-019-0232-1, 2015. a
Kulkarni, S., Deo, M. C., and Ghosh, S.: Framework for assessment of climate change impact on offshore wind energy, Meteorol. Appl., 25, 94–104, https://doi.org/10.1002/met.1673, 2018. a
Kumar, R. C. J. and Majid, M. A.: Renewable energy for sustainable development in India: current status, future prospects, challenges, employment, and investment opportunities, Energy, Sustainability and Society, 10, 1–36, https://doi.org/10.1186/s13705-019-0232-1, 2020. a
Leahy, P. G. and McKeogh, E. J.: Persistence of low wind speed conditions and implications for wind power variability, Wind Energy, 16, 575–586, https://doi.org/10.1002/we.1509, 2013. a
Lee, J.-Y., Wang, B., Wheeler, M. C., Fu, X., Waliser, D. E., and Kang, I.-S.: Real-time multivariate indices for the boreal summer intraseasonal oscillation over the Asian summer monsoon region, Clim. Dynam., 40, 493–509, https://doi.org/10.1007/s00382-012-1544-4, 2013. a
Lee, S.-S., Wang, B., Waliser, D. E., Neena, J. M., and Lee, J.-Y.: Predictability and prediction skill of the boreal summer intraseasonal oscillation in the Intraseasonal Variability Hindcast Experiment, Clim. Dynam., 45, 2123–2135, https://doi.org/10.1007/s00382-014-2461-5, 2015. a, b
Liang, Y., Fedorov, A. V., and Haertel, P.: Intensification of Westerly Wind Bursts Caused by the Coupling of the Madden-Julian Oscillation to SST During El Niño Onset and Development, Geophys. Res. Lett., 48, e2020GL089395, https://doi.org/10.1029/2020GL089395, 2021. a
Lu, T., Sherman, P., Chen, X., Chen, S., Lu, X., and McElroy, M.: India's potential for integrating solar and on- and offshore wind power into its energy system, Nat. Commun., 11, 4750, https://doi.org/10.1038/s41467-020-18318-7, 2020. a, b
Mahtta, R., Joshi, P. K., and Jindal, A. K.: Solar power potential mapping in India using remote sensing inputs and environmental parameters, Renew. Energ., 71, 255–262, https://doi.org/10.1016/j.renene.2014.05.037, 2014. a
Matsuo, Y., Endo, S., Nagatomi, Y., Shibata, Y., Komiyama, R., and Fujii, Y.: Investigating the economics of the power sector under high penetration of variable renewable energies, Appl. Energ., 267, 113956, https://doi.org/10.1016/j.apenergy.2019.113956, 2020. a
Neal, R., Robbins, J., Dankers, R., Mitra, A., Jayakumar, A., Rajagopal, E. N., and Adamson, G.: Weather pattern definitions for India and their daily historical classifications (1979 to 2016), PANGAEA [dataset publication series], https://doi.org/10.1594/PANGAEA.902030, 2019. a
Neal, R., Robbins, J., Dankers, R., Mitra, A., Jayakumar, A., Rajagopal, E. N., and Adamson, G.: Deriving optimal weather pattern definitions for the representation of precipitation variability over India, Int. J. Climatol., 40, 342–360, https://doi.org/10.1002/joc.6215, 2020. a, b, c, d, e, f, g, h
Neal, R., Guentchev, G., Arulalan, T., Robbins, J., Crocker, R., Mitra, A., and Jayakumar, A.: The application of predefined weather patterns over India within probabilistic medium-range forecasting tools for high-impact weather, Meteorol. Appl., 29, e2083, https://doi.org/10.1002/met.2083, 2022. a, b, c, d
Ohlendorf, N. and Schill, W.-P.: Frequency and duration of low-wind-power events in Germany, Environ. Res. Lett., 15, 084045, https://doi.org/10.1088/1748-9326/ab91e9, 2020. a, b, c
Otero, N., Martius, O., Allen, S., Bloomfield, H., and Schaefli, B.: A copula-based assessment of renewable energy droughts across Europe, Renew. Energ., 201, 667–677, https://doi.org/10.1016/j.renene.2022.10.091, 2022. a, b
Pryor, S. C., Letson, F. W., and Barthelmie, R. J.: Variability in Wind Energy Generation across the Contiguous United States, J. Appl. Meteorol. Clim., 59, 2021–2039, https://doi.org/10.1175/JAMC-D-20-0162.1, 2020. a
Rani, S. I., Arulalan, T., George, J. P., Rajagopal, E. N., Renshaw, R., Maycock, A., Barker, D. M., and Rajeevan, M.: IMDAA: High-Resolution Satellite-Era Reanalysis for the Indian Monsoon Region, J. Climate, 34, 5109–5133, https://doi.org/10.1175/JCLI-D-20-0412.1, 2021. a
Raynaud, D., Hingray, B., François, B., and Creutin, J. D.: Energy droughts from variable renewable energy sources in European climates, Renew. Energ., 125, 578–589, https://doi.org/10.1016/j.renene.2018.02.130, 2018. a, b, c, d, e
Rinaldi, K. Z., Dowling, J. A., Ruggles, T. H., Caldeira, K., and Lewis, N. S.: Wind and Solar Resource Droughts in California Highlight the Benefits of Long-Term Storage and Integration with the Western Interconnect, Environ. Sci. Technol., 55, 6214–6226, https://doi.org/10.1021/acs.est.0c07848, 2021. a, b
Seyedhashemi, H., Hingray, B., Lavaysse, C., and Chamarande, T.: The Impact of Low-Resource Periods on the Reliability of Wind Power Systems for Rural Electrification in Africa, Energies, 14, 2978, https://doi.org/10.3390/en14112978, 2021. a
Vitart, F.: Madden–Julian Oscillation prediction and teleconnections in the S2S database, Q. J. Roy. Meteor. Soc., 143, 2210–2220, https://doi.org/10.1002/qj.3079, 2017. a, b, c, d
Wheeler, M. C. and Hendon, H. H.: An All-Season Real-Time Multivariate MJO Index: Development of an Index for Monitoring and Prediction, Mon. Weather Rev., 132, 1917–1932, https://doi.org/10.1175/1520-0493(2004)132<1917:AARMMI>2.0.CO;2, 2004. a
White, C. J., Carlsen, H., Robertson, A. W., Klein, R. J. T., Lazo, J. K., Kumar, A., Vitart, F., Coghlan de Perez, E., Ray, A. J., Murray, V., Bharwani, S., MacLeod, D., James, R., Fleming, L., Morse, A. P., Eggen, B., Graham, R., Kjellstrom, E., Becker, E., Pegion, K. V., Holbrook, N. J., McEvoy, D., Depledge, M., Perkins-Kirkpatrick, S., Brown, T. J., Street, R., Jones, L., Remenyi, T. A., Hodgson-Johnston, I., Buontempo, C., Lamb, R., Meinke, H., Arheimer, B., and Zebiak, S. E.: Potential applications of subseasonal-to-seasonal (S2S) predictions, Meteorol. Appl., 24, 315–325, 2017. a
Wiel, K. v. d., Bloomfield, H. C., Lee, R. W., Stoop, L. P., Blackport, R., Screen, J. A., and Selten, F. M.: The influence of weather regimes on European renewable energy production and demand, Environ. Res. Lett., 14, 094010, https://doi.org/10.1088/1748-9326/ab38d3, 2019. a