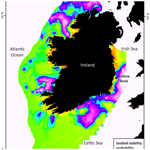
Geological seabed stability model for informing Irish offshore renewable energy opportunities
Jared L. Peters
Felix Butschek
Ross O'Connell
Valerie Cummins
Jimmy Murphy
Andrew J. Wheeler
Climate change has driven the European Union to propose a reduction in carbon emissions by increasing renewable energy production. Although Ireland is rich in renewable energy, especially offshore wind resources, it is failing to reduce its annual carbon emissions. This study endeavours to improve Ireland's marine spatial planning abilities and offshore renewable energy developments by harmonising and customising a unique geological dataset for incorporation into geospatial assessments of Ireland's continental shelf. A dataset of 1858 points, including 17 new seabed samples collected at strategic sites for this study, is created and used to build a series of geospatial outputs. Data are interpolated with empirical Bayesian kriging to use variogram analyses for probabilistically interpolating coded geological values. The interpolation results are validated through leave-one-out cross-validation and combined with bespoke models of bathymetry and seabed slope using map algebra. The final model reveals areas of relative probable seabed stability based on geological and geomorphological characteristics and is shown to comport with known conditions in several locations. Results suggest that the methods and results presented here could provide useful information to future planning activities and initial site selection assessments.
- Article
(7756 KB) - Full-text XML
-
Supplement
(64 KB) - BibTeX
- EndNote
Anthropogenic climate change is a hazard to human health and society and predictions for its progression depict increasingly dire outcomes without intervention (IPCC, 2014). Research confirms the well-established scientific consensus that carbon emissions must be reduced (Pachauri et al., 2014). Thus informed, the European Union has established ambitious renewable energy goals (EU Renewable Energy Directive (2009/28/EU)) to reduce carbon emissions and mitigate climate change impacts. These goals align with a global trend of increasing renewable energy production (International Energy Agency, 2019).
Positioned downwind of the entire fetch of the Atlantic Ocean, Ireland has abundant offshore wind and wave resources (DCENR, 2014). Unfortunately, it has yet to tap much of this energy and is falling behind other European nations in terms of offshore renewables. Ireland's carbon emissions are increasing annually by ∼2.1 million t despite its goal of reducing these by one million tonnes per year (Climate Change Advisory Council, 2018). Because of these trends, current estimates project Ireland to miss its 2030 EU Effort Sharing Regulation emissions reduction target by 92 million tonnes (ibid). Clearly, additional mitigation efforts are needed, and offshore wind power generation can make a considerable contribution.
Developing offshore wind farms, or any type of energy infrastructure, requires considerable planning and data analysis, which typically include assessments of the energy resource, costs of electrical production, and various environmental factors required to mitigate development risks (e.g. Cavazzi and Dutton, 2016; Castro-Santos et al., 2019). However, geological data are not often included in these assessments despite their potential importance to project design, costs, and environmental impacts (Peters et al., 2020). This common omission probably arises from some combination of a relative paucity of geological data, incompatibilities between disparate sources of data, and a relative inability to model these data from remote measurements. Despite these putative difficulties, previous research has shown predictive modelling is feasible (e.g. Cameron and Askew, 2011; Vasquez et al., 2015). However, these projects often produce unharmonized and discontinuous data that are difficult to use in offshore development planning (e.g. Cameron and Askew, 2011). More recently, the European Marine Observation and Data Network (EMODnet) has created improved seabed models that incorporate confidence assessments (EMODnet Geology Consortium, 2019). The EMODnet outputs are impressive; however, these products are not directly compatible with overlay analyses and significant data gaps remain – especially at national scales required for marine spatial planning.
This paper presents a novel approach for relating geological data points to a regional area by modelling data rasters to improve coverage and compatibility with assessments in Geographic Information System (GIS) environments (cf. Wilson et al., 2018; Mitchell et al., 2019). The models are created largely from public data and have been designed to improve early planning and initial opportunity assessments for Irish offshore wind development; this establishes a basic methodology that is applicable to many other nations and renewable energy types, and also inform a variety of other seabed engineering projects. The rasters provide information on surficial sediment types and geomorphology that are not typically included in preliminary offshore renewable energy assessments despite the applicability of these attributes to turbine foundation designs, scour protection measures, and cable routes (e.g. Kallehave et al., 2015). Furthermore, these assessments may improve predictions on benthic habitat changes that can be incorporated into site selection models, making preliminary examinations more compatible with environmental impact assessments.
2.1 Study area
The study area investigated for this research covers most of Ireland's continental shelf (Fig. 1). The following boundaries defined the study area: the national exclusive marine zone; the 200 m isobath; and a maximum of 110 nm from shore. These boundaries were selected based on assumptions (informed by expert opinions from academic and industry experts) that at depths >200 m and distances >110 nm offshore, the requisite technical challenges would likely render wind energy unviable, at least for the foreseeable future. The resultant area covers over ∼131 707 km2, making this a regional-scale model that is also capable of informing national marine spatial planning efforts.
2.2 Data mining, collection and treatment
Most of the data used in this research (1495 sediment sample descriptions) were provided by the Marine Institute and Geological Survey of Ireland (INFOMAR, 2020). The primary type of sedimentary data used was 7-point folk grain size descriptions, which provided sufficient detail for the goals of this research while helping to maximise data compatibility. Other data points were sourced from peer-reviewed literature on a variety of studies in or near the study area. Grab samples and vibrocores were also collected for this research during three research cruises at locations with data gaps that were likely to add information to potentially suitable areas for offshore renewable energy development (i.e. near large ports and/or population centres and <30 nm from shore). In areas without datapoints provided by the aforementioned sources, a series of estimates were made using bathymetry, backscatter, and 250 m resolution EMODnet substrate and habitat models (EMODnet Geology Consortium, 2019) at 25 km intervals (green triangles, Fig. 1). Approximations of the most likely sediment characteristics were made manually at these points by relating characteristics from the aforementioned data sources to previously established relationships between seabed morphology, hardness and modelled habitats. Many of these estimates were made outside of the study area to reduce “edging” during subsequent interpolations.
After the data points were compiled into one set, codes were assigned for sediment properties based on available information (lithological descriptions, grain size estimates, and acquisition notes or “deck sheets”). Three attributes were coded: (1) a modified 7-point Folk scale lithology descriptor; (2) a critical bed shear stress (τc) value; and (3) an angle of repose. The 7-point Folk scale was chosen as the most consistently reported and standardised metric in the available underlying sedimentary data and was used to guide inferences on the most likely τc and angle of repose. τc was adopted as a corollary to general sediment mobility based on long-established hydrological and fluvial principles (e.g. Wiberg and Smith, 1987). While grain size is not the singular factor in τc, mean sediment size and modality are major contributors to sediment mobilisation (Wilcock, 1993). Furthermore, previous research has revealed reliable relationships between grain-size data and simulated shear stress at smaller scales on the Irish seabed (Ward et al., 2015; Coughlan et al., 2019). Thus, particle size was inferred to be a goal-appropriate corollary to τc which was adopted as a general sediment mobility predictor. Value estimates for coding τc were collected from several sources (Table 1). Angle of repose is defined for this study as the steepest angle the seabed sediment is likely to maintain before slope failure from gravity-driven mass wasting and has a well-established correlation with grain size (e.g. Miller and Byrne, 1966); although this attribute is governed by many factors (e.g. grain angularity, sorting and rotation; Miller and Byrne, 1966; Yang et al., 2009), value estimates for coding this sediment property were related to grain size using industrial and academic sources (Table 1). For all attributes, higher numbers were coded for perceived (relative) positive conditions for stability (i.e. higher numbers = higher potential seabed stability). Thereby creating conditions for an “opportunities model,” rather than an “exclusions model.”
Table 1Coded sediment properties.
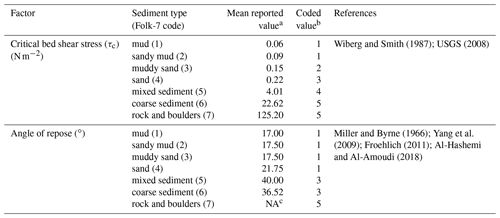
a Calculated from values compiled from the reviewed literature cited in
the “Reference” column.
b Coded for interpolation in a GIS. Values were adjusted to higher
numbers if the sediment was reported as consolidated or “stiff,”
especially for mud facies.
c No reference for rock or boulders was found for angle of repose; a
large value was assumed because rock outcrops can maintain stability at
extremely steep angles depending on composition and structure and logic
dictates that lithified grains can maintain steeper angles than loose ones.
2.3 Interpolation and validation
Full coverage rasters of the geological data were created at 500 m2 resolution through geostatistical interpolation by Empirical Bayesian Kriging (EBK) using ESRI's ArcGIS software version 10.6 following data screening to determine if the data needed to be transformed before it was kriged (Oliver and Webster, 2014). Kriging was used because it is a probabilistic, not deterministic, geostatistical interpolation method that enables cross-validation of the results (Aelion et al., 2009). EBK was specifically chosen to incorporate variographic assessments that enable subgroups of points to be interpolated independently from overall data trends (see Aelion et al., 2009; Oliver and Webster, 2014; Samsonova et al., 2017). 100 variogram models were simulated for each subset of points (100 points per subset) for all interpolations in this study.
Model validity and the appropriateness of the interpolated data were assessed using leave-one-out cross validation, which kriges the data while sequentially removing each individual datum to validate local model results. Validation outputs were assessed as the Root Mean Square Error (RMSE) and Root Mean Square Standardized Error (RMSSE) which are shown in Eqs. (1) and (2), respectively (Gaida et al., 2019).
Where n is the number of samples; is the value that has been predicted (at location xi) and z is the measured value that has been omitted at the same location.
Where σ(si) is the standard error of the prediction at site si.
2.4 Geomorphology calculations and seabed modelling
EMODnet bathymetric data were resampled to 500 m2 resolution (from ∼100 m2) and rescaled exponentially in ArcGIS to simplify the deep-water (approximately >60 m depth) seabed and emphasise the importance of shallow depths by consolidating high values there. This was done to model the increasing probability of strong currents and wave action in shallow ocean conditions. Because ocean shallowness typically correlates positively with distance to shore (i.e. depth typically increases with distance from shore), this also provides a secondary emphasis on seabed mobility from terrestrial sediment input and anthropogenic perturbations, which logically tend to converge towards shore.
Seabed slope was calculated using the geomorphometry tool package: Benthic Terrain Modeler (Walbridge et al., 2018), which computes local seabed angles using a floating neighbourhood assigned as a three-pixel by three-pixel area (Walbridge et al., 2018). The relative importance of steep slopes to seabed mobility was weighted by exponentially rescaling the slope calculations to a new raster in ArcGIS, similar to the bathymetric modelling described above.
The probability of seabed stability was modelled by summing the τc, angle of repose, modified bathymetry, and slope angles. The aforementioned rescaling functions applied to each of these rasters ensures that they cover the same range of values (1–100). Assigning identical scales to each dataset helped assure that no single aspect was spuriously weighted as more or less important to the others.
3.1 Data collection
The sedimentary data collection efforts for this research provided 1858 data points (Supplement); sources are summarised in Table 2 and all data points are shown in Fig. 1. Most data were sourced from the Marine Institute and these were typically sediment samples collected within ∼12 nm from shore (Table 2). Samples without descriptions adequate for identifying a sediment type and Folk-7 grain size were removed. Sixty-four data points were collected from 13 journal articles that were used to reduce data gaps. Additional data gaps were filled using the estimated datapoints at 25 km spacing, which resulted in 278 more data (Fig. 1; Table 2).
3.2 Interpolation
The point data were assessed for appropriateness for kriging via visual inspections of geostatistical histograms, which showed that the data were found to be distributed relatively normally with standard deviations of ∼1.3, and skewness of −0.2. Thus, no transformations were performed to reduce potential outlier effects to the interpolations and the “Power” variogram model was used to balance computational requirements and accuracy (ESRI, 2016).
This gaussian distribution also suggests that the RMSE is an appropriate method for validating of the model (Chai and Draxler, 2014). The leave-one-out cross-validation showed the EBK interpolations to be highly valid. RMSE values were all very close to zero (Table 3), indicating that interpolated values were often consistent with actual values (Arslan, 2012). Furthermore, values for the RMSSE were typically close to one, indicating validity, and most often less than one, suggesting that predictions may overestimate variability (Gaida et al., 2019). This potential overestimation is not necessarily a drawback, given the simplified characterisations of the sedimentary data required to code information for use in the model.
Table 3Mean results for all points for the leave-one-out cross-validation of the EBK interpolations used in the seabed stability model.
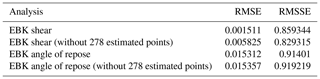
Results from the Folk-7 sediment categories are interpolated (Lark et al., 2012) and presented here (Fig. 2) despite these values not being directly used in the following seabed stability model. These interpolated values and the resulting map provide a stand-alone output that adds to previous seabed mapping efforts for Ireland (e.g. EMODnet Geology Consortium, 2019). The area covered by each interpolated sediment type is calculated in a GIS and shown in Table 4. Sand is revealed to be the most common seabed sediment (Fig. 2), covering ∼63 400 km2 of the study area (∼48 %; Table 4). Rock and muddy sediments are shown to be rare on the shallow (<200 m depth) Irish continental shelf (Table 4) and mud is mainly found in the Irish Sea “mud belt” (Belderson, 1964; Coughlan et al., 2015). Some outcrops of subglacial diamiction are discernible in the interpolated raster, at least in western Ireland, where bands of mixed sediment (ostensibly from sampled diamictic facies) can be identified roughly aligning with mapped moraines and grounding-zone wedges associated with the last British-Irish Ice Sheet (Peters et al., 2015, 2016; Fig. 2).
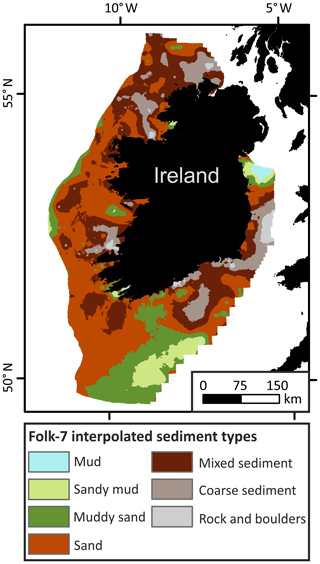
Figure 2Map of interpolated values of the coded Folk-7 sediment categories (coded values in Table 1).
Results of the EBK interpolations and raster rescaling are shown in Fig. 3. The mapped outputs of these results reveal similar data distributions for τc and angle of repose; however high values for angle of repose are less extensive. This is expected from the differences in value coding (Table 1). Clear similarities in the patterns of likely sediment stability can be seen after the initial results are rescaled (Fig. 3b, d); this was also expected given the common origin of the coded sediment properties (the sedimentary dataset shown in Fig. 1).
3.3 Seabed stability modelling
The results of the initial seabed modelling for bathymetry and slope are shown in Fig. 4. The modified bathymetric model (data were rescaled exponentially and inverted) reveals a relatively wide band of shallow water in the Irish Sea (east of Ireland) which contributes to near-shore seabed instability potential (Fig. 4b). Conversely, the Atlantic Ocean off western Ireland is characterised by deeper water, but nearshore instability is more likely from steeper seabed slopes (Fig. 4d). Megaridges in the Celtic Sea (Lockhard et al., 2018) create a series of small, steep slopes (Fig. 4c, d). However, these slopes typically do not result in as many low values (i.e. high slope angles) as the near-shore data from the bathymetric model (Fig. 4b).
These preliminary outputs (Fig. 4) are summed together with the interpolated sedimentary seabed models (Fig. 3) to estimate the overall seabed stability (Fig. 5). In this final model output, higher (unitless) values generally correspond to seabed conditions typified by coarse and/or consolidated material at low slopes and in relatively deep water. A large area of rocky and coarse-grained seabed with shallow slopes in the Celtic Sea, south of Ireland, is identified as relatively immobile. Areas interpolated from rock or boulder Folk-7 geological data, which are most often found within 12-nm of shore, are also identified as stable. This reflects the scope of this model (to predict areas of stable seabed) and highlights the need for careful interpretation in its use – predicted instability is not necessarily synonymous with unsuitable for development.
Several locations of fine-grained seabed sediments proximal to shore are identified as the most geologically unstable areas. All other variables being equal (e.g. METocean conditions, sediment influx rates, ocean currents), these areas are modelled to be most likely to experience seabed mobilisations and will likely require the greatest mitigation efforts (e.g. environmental monitoring, scour protection, dredging) if they are developed. Several lines of evidence corroborate these results. Firstly, sediment mobilisation and subsequent removal of muddy sediments in the Irish Sea has been shown to occur relatively quickly and to be exacerbated by anthropogenic activities (Coughlan et al., 2015). And secondly, dredging reports from Ireland's first (and currently only) offshore wind farm, Arklow Bank (Fig. 5), document sediment accumulation at rates that may compromise safe access to the turbines without removal (Moore et al., 2016). This suggests that sediment mobilisation was significant following installation of turbine foundations, which aligns with documented evidence (Whitehouse et al., 2011). The potential mobilisation of both the Irish Sea mud belt and sandy sediments near Arklow Bank are predicted by the final seabed stability model (Fig. 5).
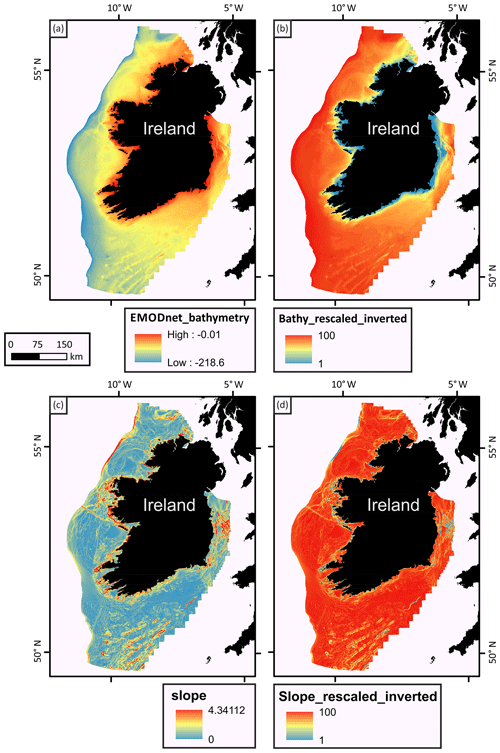
Figure 4Bathymetry and slope modelling, rescaling, and inversion results. (a) Original EMODnet bathymetric data. (b) Rescaled and inverted bathymetric data, modelled to estimate the influence of depth and distance from shore on sediment stability. (c) Slope calculation results. (d) Rescaled and inverted slope raster.
This model is a promising addition to ongoing research seeking to inform Irish marine spatial planning and site investigations for potential offshore renewable energy developments. Additionally, this work documents a promising method for collecting and interpolating various types of geological data. However, this model has been designed to be used in GIS-based multi-criteria decision analyses with other parameters to help provide more complete assessments. Furthermore, the work presented here was informed solely by bathymetry and the composition of the seabed, which are not the only factors driving sediment mobility. Thus, for example, this model cannot distinguish areas of seabed stability due to inherently stable sediments, from areas undergoing modern winnowing and active fine particle mobilisation due to local currents – which would likely lead to unstable seabed conditions following disruptions from infrastructure installation. Future work should build on this research by incorporating modelled metocean forcing data that will enable an assessment of a primary erosive mechanism that drives sediment mobilisation.
This new model of seabed stability is unique from previous efforts because it is guided by engineering aspects of offshore development for renewable energy infrastructure. Thus, sedimentary properties are used to relate the likely seabed conditions to the most pertinent aspects of sediment mobility for the installation and maintenance of foundations for renewable energy structures. These characteristics are also important for assessing the potential for environmental disturbances following foundation installations. Given the ambitious goals established by the Climate Change Advisory Council (2018) to curb Ireland's carbon emissions, this geological aid to de-risking marine development is highly relevant. No other bespoke geological tool was previously available at such a scale.
The new model presented here harmonises various seabed data (N=1858, ranging from samples to photographs) to create a bespoke dataset for assessing the Irish continental shelf for offshore renewable energy development. The compiled data are interpolated via empirical Bayesian kriging, a well-established geostatistical technique typically used in geological and environmental analyses. The interpolation results extend data coverage while rendering the dataset compatible with common geospatial assessments used in offshore site selection investigations and marine spatial planning efforts. Highlights include:
-
A novel dataset composed of 1858 data of various types, including 17 new seabed samples strategically collected for this study, is harmonised and coded for unique, regional geostatistical analyses and geospatial interpolation.
-
A new rasterised, continuous seabed map is provided that is shown to comport spatially with several known sediment types and Quaternary seabed processes. This output helps qualitatively validate the seabed stability models derived from much of the same data and provides new seabed data that may aid in future habitat mapping or planning assessments.
-
Empirical Bayesian kriging is shown to be a useful addition to geospatial assessments of offshore renewable energy potential and the results of the interpolations used for this study are shown to be mathematically valid through leave-one-out cross-validation.
-
Interpolated sediment properties reveal patterns in the probabilistic distribution of seabed stability derived from the original sedimentary dataset.
-
Models of seabed slope and bathymetry that are customised for highlighting geomorphically stable areas are presented. These models weight high angles and shallow depths exponentially to reflect the importance of these aspects in seabed stability.
-
The models of sediment properties, angle and bathymetry are summed together in a GIS to model the overall probability of seabed stability for the shallow (<200 m deep) Irish continental shelf. This map is shown to accurately highlight relative seabed instabilities in several locations and can be used in future multi-criteria decision analyses in GIS environments. This is a promising result for establishing regional or national-scale geological models that are useful for preliminary offshore renewable energy planning.
Sources for existing publicly available data are provided; information on new data presented in this study is provided in the Supplement.
The supplement related to this article is available online at: https://doi.org/10.5194/adgeo-54-55-2020-supplement.
JLP performed the conceptualisation, data curation, formal analysis, validation, visualisation, and writing. FB assisted in GIS analysis and writing. RO'C aided in conceptualisation and visualisation; AW aided in conceptualisation and provided supervision. AJW, VC, and JM acquired funding.
The authors declare that they have no conflict of interest.
This article is part of the special issue “European Geosciences Union General Assembly 2020, EGU Division Energy, Resources & Environment (ERE)”. It is a result of the EGU General Assembly 2020, 4–8 May 2020.
This study was conducted as part of the EirWind Project (https://www.marei.ie/eirwind/#tab-id-6, last access: 8 October 2020), which has received funding from the following industry partners: Brookfield Renewable Ireland, DP Energy Ireland, EDP Renewables, Electricity Supply Board, Enerco Energy, ENGIE, Equinor ASA, Simply Blue Energy, SSE Renewables, and Statkraft Ireland; Science Foundation Ireland (SFI) under Grant No 12/RC/2302 (MaREI); and University College Cork, Ireland. Additional funding was provided by the Marine Institute from Ship Time Awards: CV18034, CV19023, and CV19026. Mapping analysis was partially funded by the Science Foundation Ireland under Grant Number 13/RC/2092 and is co-funded under the European Regional Development Fund and by iCRAG industry partners (iCRAG). The Marine Institute and Geological Survey Ireland are thanked for the use of their vibrocorer. This manuscript was improved by a helpful review from Mark Coughlan.
This research has been supported by the Science Foundation Ireland (grant no. 12/RC/2302).
This paper was edited by Johannes Miocic and reviewed by Mark Coughlan and one anonymous referee.
Aelion, C. M., Davis, H. T., Liu, Y., Lawson, A. B., and McDermott, S.: Validation of Bayesian kriging of arsenic, chromium, lead, and mercury surface soil concentrations based on internode sampling, Environ. Sci. Technol., 43, 4432–4438, 2009.
Al-Hashemi, H. M. B. and Al-Amoudi, O. S. B.: A review on the angle of repose of granular materials, Powder Technology, 330, 397–417, https://doi.org/10.1016/j.powtec.2018.02.003, 2018.
Arslan, H.: Spatial and temporal mapping of groundwater salinity using ordinary kriging and indicator kriging: The case of Bafra Plain, Turkey, Agric. Water Manag., 113, 57–63, https://doi.org/10.1016/j.agwat.2012.06.015, 2012.
Belderson, R. H.: Holocene sedimentation in the western half of the Irish Sea. Mar. Geol., 2, 147–163, 1964, https://doi.org/10.1016/0025-3227(64)90032-5.
British Geological Survey: Geoindex Offshore, available at: http://mapapps2.bgs.ac.uk/geoindex_offshore/home.html?cruise=1968/1 (last access: 8 October 2020), contains British Geological Survey materials © NERC, 2020.
Callard, S. L., Ó Cofaigh, C., Benetti, S., Chiverrell, R. C., Van Landeghem, K. J., Saher, M. H., Gales, J. A., Small, D., Clark, C., Livingstone, S. J., Fabel, D., and Moreton, S. G.: Extent and retreat history of the Barra Fan Ice Stream offshore western Scotland and northern Ireland during the last glaciation, Quaternary Sci. Rev., 201, 280–302, https://doi.org/10.1016/j.quascirev.2018.10.002, 2018.
Callard, S. L., Cofaigh, C. Ó., Benetti, S., Chiverrell, R. C., Van Landeghem, K. J., Saher, M. H., Livingstone, S. J., Clark, C., Small, D., Fabel, D., and Moreton, S. G.: Oscillating retreat of the last British-Irish Ice Sheet on the continental shelf offshore Galway Bay, western Ireland, Mar. Geol., 420, 106087, https://doi.org/10.1016/j.margeo.2019.106087, 2020.
Cameron, A. and Askew, N.: EUSeaMap – Preparatory Action for development and assessment of a European broad-scale seabed habitat map final report, available at: http://jncc.gov.uk/euseamap (last access: 8 October 2020), 2011.
Castro-Santos, L., Garcia, G. P., Simões, T., and Estanqueiro, A.: Planning of the installation of offshore renewable energies: A GIS approach of the Portuguese roadmap, Renew. Energy, 132, 1251–1262, https://doi.org/10.1016/j.renene.2018.09.031, 2019.
Cavazzi, S. and Dutton, A. G.: An Offshore Wind Energy Geographic Information System (OWE-GIS) for assessment of the UK's offshore wind energy potential, Renew. Energy, 87, 212–228, https://doi.org/10.1016/j.renene.2015.09.021, 2016.
Chai, T. and Draxler, R. R.: Root mean square error (RMSE) or mean absolute error (MAE)? – Arguments against avoiding RMSE in the literature, Geosci. Model Dev., 7, 1247–1250, https://doi.org/10.5194/gmd-7-1247-2014, 2014.
Climate Change Advisory Council: Annual Review 2018, Report, ISBN 978-1-84095-788-4, available at: http://www.climatecouncil.ie/media/CCAC_AnnualReview2018.pdf, last access: 24 August 2020.
Coughlan, M., Wheeler, A. J., Dorschel, B., Lordan, C., Boer, W., Van Gaever, P., De Haas, H., and Mörz, T.: Record of anthropogenic impact on the Western Irish Sea mud belt, Anthropocene, 9, 56–69, https://doi.org/10.1016/j.ancene.2015.06.001, 2015.
Coughlan, M., Guerrini, M., Creane, S., Musialik, M., O'Shea, M., and Murphy, J.: A Morphodynamic Study of the Irish Sector of the Irish Sea, Wind Europe, Marine Institute Grant No. IND/18/18, 1–10, Wind Europe: Offshore 2019, Copenhagen, 26–28 November 2019.
DCENR (Department of Communications Energy and Natural Resources): Offshore Renewable Energy Development Plan (Issue February), Report, available at: https://www.dccae.gov.ie/en-ie/energy/topics/Renewable-Energy/electricity/offshore/offshore-renewable-energy-development-plan-/Pages/Offshore-Renewable-Energy-Development-Plan.aspx (last access: 24 August 2020), 2014.
Delivet, S., Van Eetvelt, B., Monteys, X., Ribó, M., and Van Rooij, D.: Seismic geomorphological reconstructions of Plio-Pleistocene bottom current variability at Goban Spur, Mar. Geol., 378, 261–275, https://doi.org/10.1016/j.margeo.2016.01.001, 2016.
EMODnet Geology Consortium: EMODnet Geology Phase 3 – Final Report, available at: https://www.emodnet-geology.eu/trimonthly-reports/ (last access: 8 October 2020), 2019.
ESRI: What is Empirical Bayesian kriging?, available at: https://desktop.arcgis.com/en/arcmap/10.3/guide-books/extensions/geostatistical-analyst/what-is-empirical-bayesian-kriging-.htm (last access: 2 May 2020), 2016.
Froehlich, D. C.: Mass angle of repose of open-graded rock riprap, J. Irrig. Drain, E., 137, 454–461, https://doi.org/10.1061/(ASCE)IR.1943-4774.0000316, 2011.
Gaida, T. C., Snellen, M., van Dijk, T. A., and Simons, D. G.: Geostatistical modelling of multibeam backscatter for full-coverage seabed sediment maps, Hydrobiologia, 845, 55–79, https://doi.org/10.1007/s10750-018-3751-4, 2019.
Heindel, K., Titschack, J., Dorschel, B., Huvenne, V. A., and Freiwald, A.: The sediment composition and predictive mapping of facies on the Propeller Mound – A cold-water coral mound (Porcupine Seabight, NE Atlantic), Cont. Shelf Res., 30, 1814–1829, https://doi.org/10.1016/j.csr.2010.08.007, 2010.
Huvenne, V. A. I., Blondel, P., and Henriet, J. P.: Textural analyses of sidescan sonar imagery from two mound provinces in the Porcupine Seabight, Mar. Geol., 189, 323–341, https://doi.org/10.1016/S0025-3227(02)00420-6, 2002.
INFOMAR: Web Services, available at: https://www.infomar.ie/services, last access: 26 April 2020.
International Energy Agency: Renewables 2019, IEA, Paris, available at: https://www.iea.org/reports/renewables-2019 (last access: 8 October 2020), 2019.
IPCC: Summary for policymakers In: Climate Change 2014: Impacts, Adaptation, and Vulnerability. Part A: Global and Sectoral Aspects, Contribution of Working Group II to the Fifth Assessment Report of the Intergovernmental Panel on Climate Change, Cambridge University Press, Cambridge Cambridge, United Kingdom and New York, NY, USA, edited by: Field, C. B., Barros, V. R., Dokken, D. J., Mach, K. J. Mastrandrea,, M. D., Bilir, T. E., Chatterjee, M., Ebi, K. L., Estrada, Y. O., Genova, R. C., Girma, B., Kissel, E. S., Levy, A. N., MacCracken, S., Mastrandrea, P. R., and White, L. L., pp. 1–32, 2014.
Kallehave, D., Byrne, B. W., LeBlanc Thilsted, C., and Mikkelsen, K. K.: Optimization of monopiles for offshore wind turbines, Philos. Trans. R. Soc. A., 373, 20140100, https://doi.org/10.1098/rsta.2014.0100, 2015.
Lark, R. M., Dove, D., Green, S. L., Richardson, A. E., Stewart, H., and Stevenson, A.: Spatial prediction of seabed sediment texture classes by cokriging from a legacy database of point observations, Sediment. Geol., 281, 35–49, https://doi.org/10.1016/j.sedgeo.2012.07.009, 2012.
Lim, A., Wheeler, A. J., and Arnaubec, A.: High-resolution facies zonation within a cold-water coral mound: The case of the Piddington Mound, Porcupine Seabight, NE Atlantic, Mar. Geol., 390, 120–130, https://doi.org/10.1016/j.margeo.2017.06.009, 2017.
Lockhart, E. A., Scourse, J. D., Praeg, D., Van Landeghem, K. J., Mellett, C., Saher, M., Callard, L., Chiverrell, R. C., Benetti, S., Ó Cofaigh, C., and Clark, C. D.: A stratigraphic investigation of the Celtic Sea megaridges based on seismic and core data from the Irish-UK sectors, Quaternary Sci. Rev., 198, 156–170, https://doi.org/10.1016/j.quascirev.2018.08.029, 2018.
Miller, R. L. and Byrne, R. J.: The angle of repose for a single grain on a fixed rough bed, Sedimentology, 6, 303–314, https://doi.org/10.1111/j.1365-3091.1966.tb01897.x, 1966.
Mitchell, P. J., Aldridge, J., and Diesing, M.: Legacy data: how decades of seabed sampling can produce robust predictions and versatile products, Geosciences, 4, 182, https://doi.org/10.3390/geosciences9040182, 2019.
Moore, K., Emberton, R., and Royall, M.: Arklow Bank dumping at sea permit application supporting information, 1620000345-002/1, 1–36, Ramboll Environ, Southampton, UK, available at: http://www.epa.ie/terminalfour/DaS/DaS-view.jsp?regno=S0027-01 (last access: 8 October 2020), 2016.
Oliver, M. A. and Webster, R.: A tutorial guide to geostatistics: Computing and modelling variograms and kriging, Catena, 113, 56–69, 2014.
Pachauri, R. K., Allen, M. R., Barros, V. R., Broome, J., Cramer, W., Christ, R., Church, J. A., Clarke, L., Dahe, Q., Dasgupta, P., Dubash, N. K., Edenhofer, O., Elgizouli, I., Field, C. B., Forster, P., Friedlingstein, P., Fuglestvedt, J., Gomez-Echeverri, L., Hallegatte, S., Hegerl, G., Howden, M., Jiang, K., Jimenez Cisneroz, B., Kattsov, V., Lee, H., Mach, K. J., Marotzke, J., Mastrandrea, M. D., Meyer, L., Minx, J., Mulugetta, Y., O'Brien, K., Oppenheimer, M., Pereira, J. J., Pichs-Madruga, R., Plattner, G. K., Pörtner, H. O., Power, S. B., Preston, B., Ravindranath, N. H., Reisinger, A., Riahi, K., Rusticucci, M., Scholes, R., Seyboth, K., Sokona, Y., Stavins, R., Stocker, T. F., Tschakert, P., van Vuuren, D., and van Ypserle, J. P.: Climate Change 2014: Synthesis Report, Contribution of Working Groups I, II and III to the Fifth Assessment Report of the Intergovernmental Panel on Climate Change, edited by: Pachauri, R. and Meyer, L., Geneva, Switzerland, IPCC, 151 p., ISBN 978-92-9169-143-2, 2014.
Peters, J. L., Benetti, S., Dunlop, P., and Ó Cofaigh, C.: Maximum extent and dynamic behaviour of the last British–Irish Ice Sheet west of Ireland, Quaternary Sci. Rev., 128, 48–68, https://doi.org/10.1016/j.quascirev.2015.09.015, 2015.
Peters, J. L., Benetti, S., Dunlop, P., Ó Cofaigh, C., Moreton, S. G., Wheeler, A. J., and Clark, C. D.: Sedimentology and chronology of the advance and retreat of the last British-Irish Ice Sheet on the continental shelf west of Ireland, Quaternary Sci. Rev., 140, 101–124, https://doi.org/10.1016/j.quascirev.2016.03.012, 2016.
Peters, J. P., Remmers, T., Wheeler, A. J., Murphy, J., and Cummins, V.: A systematic review and meta-analysis of GIS use to reveal trends in offshore wind energy research and offer insights on best practices, Renew. Sust. Energ. Rev., 128, 109916, https://doi.org/10.1016/j.rser.2020.109916, 2020.
Raes, M. and Vanreusel, A.: Microhabitat type determines the composition of nematode communities associated with sediment-clogged cold-water coral framework in the Porcupine Seabight (NE Atlantic), Deep-Sea Res. Pt. I, 53, 1880–1894, https://doi.org/10.1016/j.dsr.2006.08.012, 2006.
Samsonova, V. P., Blagoveshchenskii, Y. N., and Meshalkina, Y. L.: Use of empirical Bayesian kriging for revealing heterogeneities in the distribution of organic carbon on agricultural lands, Eurasian Soil Sci+, 50, 305–311, https://doi.org/10.1134/S1064229317030103, 2017.
Scourse, J., Saher, M., Van Landeghem, K. J., Lockhart, E., Purcell, C., Callard, L., Roseby, Z., Allinson, B., Pieńkowski, A. J., Ó Cofaigh, C., Praeg, D., Ward, S., Chiverrell, R., Moreton, S., Fabel, D., and Clark, C.: Advance and retreat of the marine-terminating Irish Sea Ice Stream into the Celtic Sea during the Last Glacial: Timing and maximum extent, Mar. Geol., 412, 53–68, https://doi.org/10.1016/j.margeo.2019.03.003, 2019.
Somerfield, P. J., McClelland, I. L., McNeill, C. L., Bolam, S. G., and Widdicombe, S.: Environmental and sediment conditions, infaunal benthic communities and biodiversity in the Celtic Sea, Cont. Shelf Res., 185, 23–30, https://doi.org/10.1016/j.csr.2018.09.002, 2019.
USGS (United States Geological Survey): Scientific Investigations Report 2008–5093, available at: http://pubs.usgs.gov/sir/2008/5093 (last access: 8 October 2020), 2008.
Van Rooij, D., De Mol, B., Huvenne, V., Ivanov, M., and Henriet, J. P.: Seismic evidence of current-controlled sedimentation in the Belgica mound province, upper Porcupine slope, southwest of Ireland, Mar. Geol., 195, 31–53, https://doi.org/10.1016/S0025-3227(02)00681-3, 2003.
Vasquez, M., Chacón, D.M., Tempera, F., O'Keeffe, E., Galparsoro, I., Alonso, J.S., Gonçalves, J.M., Bentes, L., Amorim, P., Henriques, V., and McGrath, F.: Broad-scale mapping of seafloor habitats in the north-east Atlantic using existing environmental data, J. Sea Res., 100, 120–132, https://doi.org/10.1016/j.seares.2014.09.011, 2015.
Walbridge, S., Slocum, N., Pobuda, M., and Wright, D. J.: Unified Geomorphological Analysis Workflows with Benthic Terrain Modeler, Geosci. J., 8, 94, https://doi.org/10.3390/geosciences8030094, 2018.
Ward, S. L., Neill, S. P., Van Landeghem, K. J., and Scourse, J. D.: Classifying seabed sediment type using simulated tidal-induced bed shear stress, Mar. Geol., 367, 94–104, https://doi.org/10.1016/j.margeo.2015.05.010, 2015.
Whitehouse, R. J., Harris, J. M., Sutherland, J., and Rees, J.: The nature of scour development and scour protection at offshore windfarm foundations, Mar. Pollut. Bull., 62, 73–88, https://doi.org/10.1016/j.marpolbul.2010.09.007, 2011.
Wiberg, P. L. and Smith, J. D.: Calculations of the critical shear stress for motion of uniform and heterogeneous sediments, Water Resour. Res., 23, 1471–1480, 1987.
Wilcock, P. R.: Critical shear stress of natural sediments, J. Hydraul. Eng., 119, 491–505, 1993.
Wilson, R. J., Speirs, D. C., Sabatino, A., and Heath, M. R.: A synthetic map of the north-west European Shelf sedimentary environment for applications in marine science, Earth Syst. Sci. Data, 10, 109–130, https://doi.org/10.5194/essd-10-109-2018, 2018.
Yang, F. G., Liu, X. N., Yang, K. J., and Cao, S. Y.: Study on the angle of repose of nonuniform sediment, J. Hydrodynam., 21, 685–691, https://doi.org/10.1016/S1001-6058(08)60200-0, 2009.